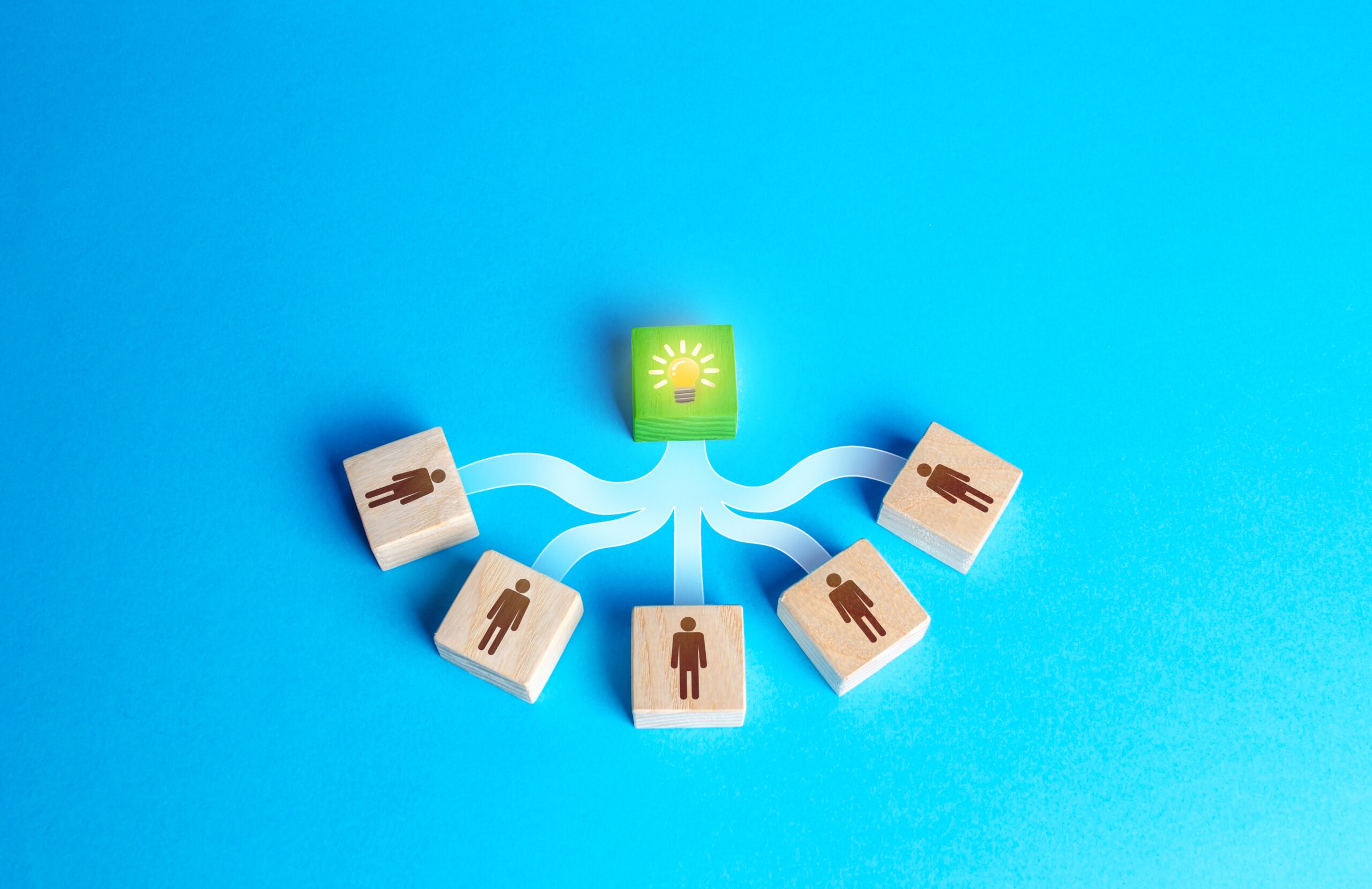
Data Lineage: The Backbone of Responsible AI Decision-Making
BSI – 04/04/2025
In the rapidly evolving world of artificial intelligence, transparency and accountability are no longer optional—they’re essential. As AI systems increasingly influence critical business decisions across industries, it becomes vital to ensure that every decision made by a model can be traced back to the data it was trained on. This is where data lineage plays a transformative role.
Data lineage is the life story of data—where it came from, how it was transformed, and where it went. It’s the visual or metadata-driven tracking of data flow across systems. I
How AI Makes Decisions
Artificial Intelligence (AI) makes decisions by analyzing data, identifying patterns, and choosing the most appropriate action based on its training and objectives. The process starts with input data, such as text, images, transactions, or sensor readings, which is cleaned and transformed into a format the AI model can understand.
Once prepared, the data is fed into a trained model that has learned from large amounts of historical information. This model evaluates the inputs using statistical relationships and predictive logic to produce an output. The output could be a classification (e.g., “fraud” or “not fraud”), a prediction (e.g., likelihood of loan repayment), or a recommended action (e.g., approve a claim).
AI decisions are guided by predefined goals such as accuracy, efficiency, risk reduction, or user satisfaction. In more advanced setups, AI systems can learn over time, adapt to new data, and even reason through multi-step problems, especially in the case of agentic or autonomous AI.
While some decisions are fully automated, others involve a human-in-the-loop for oversight and accountability, especially in critical areas like healthcare, finance, and insurance.
What is Data Lineage?
Data lineage refers to the lifecycle of data—how it moves through systems, transforms, and evolves from its original source to its final form in decision-making processes. In the context of AI, it means maintaining a clear trail from raw data inputs all the way to model outputs and predictions.
Imagine a customer churn prediction model used by a telecom company. Data lineage enables the company to track exactly which customer behavior metrics—like call drop rates, usage patterns, or service complaints—contributed to a specific churn prediction. This traceability builds trust, helps ensure compliance, and allows businesses better to explain AI-driven actions to stakeholders and regulators alike.
Why Data Lineage Matters in AI
At Business Software India, we believe that AI should not be a black box. Responsible AI requires models that are not only performant but also auditable, explainable, and trustworthy. Here’s why data lineage is critical in this mission:
- Transparency and Trust
When businesses and users can see how a decision was made and what data influenced it, they are more likely to trust AI systems. This transparency becomes a competitive advantage, particularly in regulated sectors like finance, healthcare, and legal services. - Debugging and Quality Control
If a model starts making unexpected predictions, data lineage helps engineers quickly identify whether the issue stems from corrupted input data, transformation errors, or model drift. This significantly reduces downtime and improves reliability. - Regulatory Compliance
Data lineage ensures companies can respond to audits, fulfill data subject rights requests, and demonstrate compliance with minimal friction. - Model Improvement and Retraining
Understanding how specific data points affect model performance allows for better fine-tuning. It provides a feedback loop that continuously improves both model accuracy and fairness.
Example: Data Lineage in Insurance
Let’s say you’re building an automated risk scoring system for insurance claims:
So if a claim is flagged as “high risk”, lineage shows:
- Who entered the original customer data
- What transformations were applied
- Which version of the ML model was used
- Where and how the decision was presented to the adjuster
Our Approach at Business Software India
At Business Software India, we embed data lineage tracking into every stage of our AI model development pipeline. Our AI platforms are designed to:
- Record metadata about data sources and preprocessing steps
- Track changes in training data over time
- Log model versions, training parameters, and input-output mappings
- Provide audit trails for each decision made by a deployed model
This disciplined approach allows our clients to build AI systems that are explainable, resilient, and ethically sound—all while aligning with business goals and industry regulations.
As India’s digital economy continues to grow, so does the responsibility to build AI systems that serve society fairly and transparently. Data lineage isn’t just a technical feature—it’s a commitment to ethical AI development. At Business Software India, we’re proud to lead by example, delivering solutions that are not only intelligent but also accountable.